IKnife4Foam
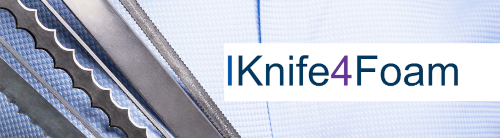
Project Description
The investigations took place within the framework of the R&D project IKnife4Foam in cooperation with Albrecht Bäumer GmbH & Co. KG (AB). The project was kindly funded by the Central Innovation Program for SMEs (ZIM) of the Federal Ministry for Economic Affairs and Energy (BMWi) under the number 16KN080127. It is focused on condition monitoring and predictive maintenance of contour cutting machines for foam block processing. These machines typically use an endless, circumferential band blade that is guided and driven by multiple pulleys and continuously moves through a cutting area to facilitate precise 3D-cutting operations (Fig. 1). The developed methodology and system are designed to detect imminent band blade failures and to predict the remaining lifetime of the blades. The project entails the meticulous selection and development of sensor technology and advanced data evaluation algorithms tailored for this specific application. These algorithms leverage machine learning techniques, incorporating features derived from the physical attributes of the band blade, machine kinematics, and operational parameters. The primary objective of this innovative system is to facilitate proactive maintenance by predicting blade failures in advance, thereby mitigating unplanned downtime associated with blade replacements. The system enables strategic, condition-based maintenance scheduling by providing a reliable prognosis of remaining useful life. The developed solution not only enhances productivity but also leads to less waste and thus contributes to environmental protection because the band blade usually breaks during the cutting process, and the foam block being processed then has to be scrapped or at least partially scrapped. Another important advantage is increased safety, as employees no longer have to remove the sharp scrap parts of the broken band blade from the machine in case of breakage. Furthermore, it promotes sustainable practices by optimizing the utilization of new band blades. Ultimately, this technological advancement positions Albrecht Bäumer as a frontrunner in the market, offering a distinct competitive edge over industry rivals.
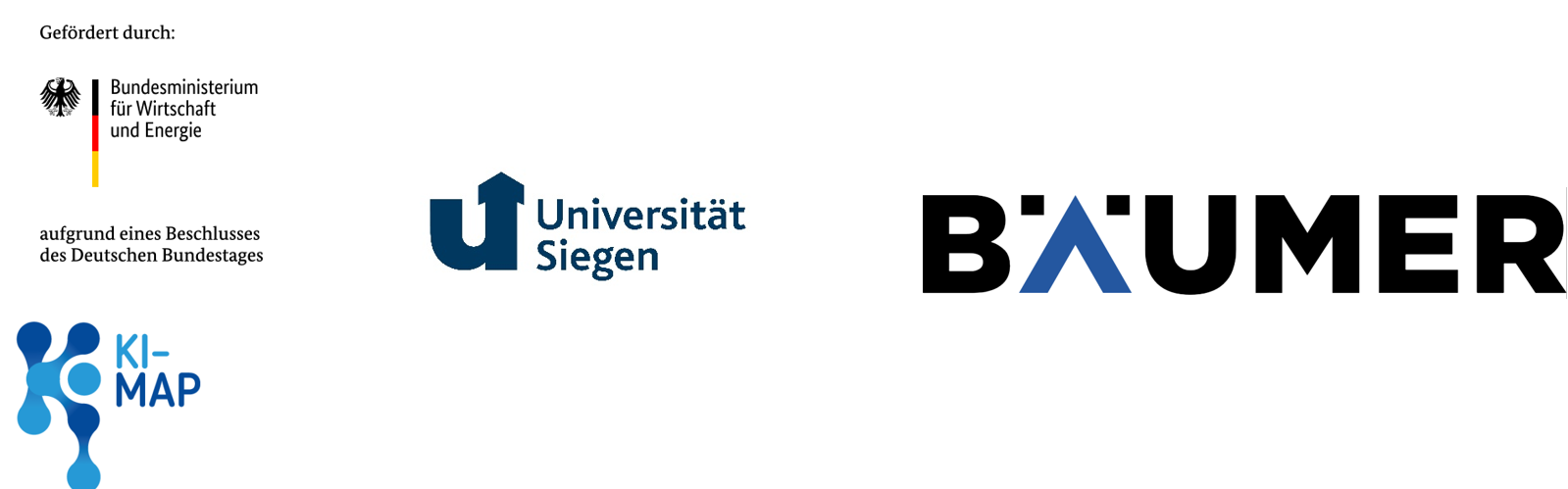
Outlook
The IKnife4Foam project, led by Albrecht Bäumer GmbH & Co. KG and the University of Siegen (Chair "Mechanics with focus Structural-Health-Monitoring" - MSHM), successfully finished and completed its aim of detecting band blade failures in contour cutting machines on 31.10.2023. This was achieved through the development of various numerical models, extensive sensor-based tests, and prognostic models. Additionally, specialized equipment was developed for realistic blade failure tests and static blade analysis. One of the main challenges we faced was the constant high-speed rotation of the band blades with 14m/s, which made traditional sensor technologies inadequate. To overcome this, we used contact and non-contact sensors to extract blade health features while the blade moved rapidly. Our project focused on the OFS-HE3 contour cutting machine, which utilizes a rotating horizontal blade (refer to Figure 1). As part of this R&D project, we have developed a prognostic model for this machine. Additionally, the model can be extended to other similar contour-cutting machines.
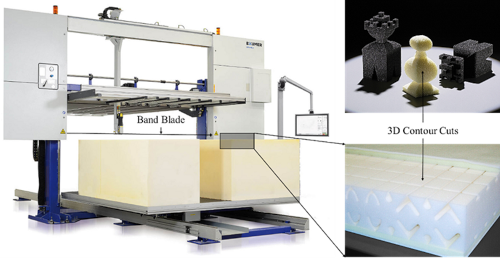
Experimental Setup
Albrecht Bäumer (AB) designed and manufactured a modified so called segment machine based on the OFS-HE3. The machine was installed at the University of Siegen in collaboration with AB. Its purpose is to perform accelerated run-to-failure tests while simulating various load conditions, cutting programs and cutting speeds. During the project period, we monitored two additional machines: one located at AB's technical centre and another in a realistic production environment located at a customer of AB.
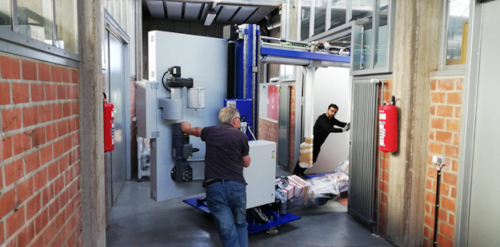
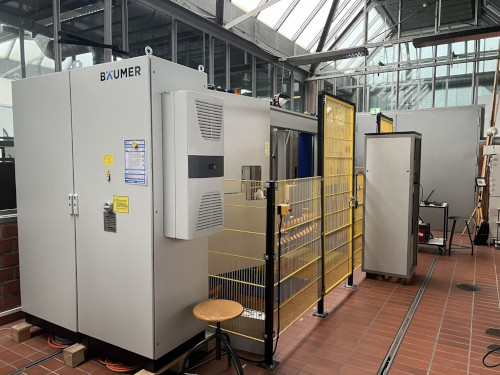
The integration of sensors into contour cutting machines has enabled real-time monitoring and data collection under various operating conditions. Preliminary tests were conducted to optimize sensor location and count. Based on these results, we identified different band blade failure locations and the machine's critical points. Sensors were then strategically placed at these locations to monitor different failure mechanisms. Various sensors were utilized during the tests to measure blade failure propagation across different frequencies. These included strain gauges, acceleration sensors, temperature sensors, 1D laser sensors, acoustic emission sensors, and eddy current sensors. Furthermore, parameters from the machine servo drives were closely monitored. During operation at speeds of 14m/s, various high-speed cameras were used to monitor failure locations of the band blade and detect possible cracks forming on the band.
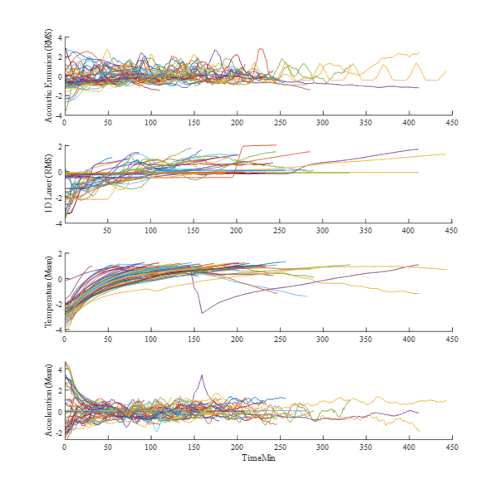
Finite Element Modelling of Band Blade
As part of the project, we conducted a series of multi-part finite element analyses to better understand the band blade's damage mechanics. These tests also helped us optimize the sensor positions for the initial tests. The FEM model we used represents the same dimensions and part orientation as the segment machine, with four deflection rollers and three twisting units. In order to achieve a stable model, we needed to consider various factors such as model settings, contact boundary constraints, and material and geometrical properties. The analysis focused on a rotating band blade. We cooperated with our project partner, AB, to perform stress-strain analyses and design the material of the band blade for the FEM. Based on the results, we were able to model both elastic and plastic material properties for the simulation model. The model is quite versatile, allowing for the variation of simulated machine parameters and testing different operating conditions.
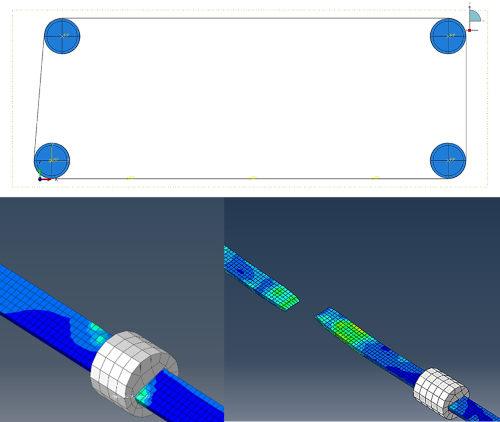
Band Blade Prognostics
Over 50 run-to-failure tests were conducted to create a comprehensive dataset of band blade failures. Machine learning models were then used to accurately estimate the service life of the blades and classify their conditions. The sensor features with the highest prognosis ability, or trend towards failure, were selected based on the measured sensor and machine values to further develop the prognostics model. By utilizing data-based machine learning models such as Similarity Models and Long Short-Term Memory Network (LSTM), a forecast of remaining service life has been achieved, along with an early fault classification model using.
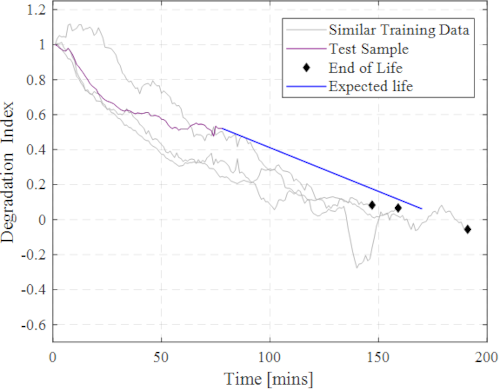
Experimental Test Branch
It has been noted that the distance between a twisting unit and a pulley is a crucial factor affecting the band knife's load and wear. As it was not possible to design the segment machine with variable spacing of these components, it was decided to create an additional test stand (Figure 7) with variable spacing. The test stand enables experimental investigation of the influence of twisting distance on the band knife. Variable spacing provides an additional means of increasing belt load, thereby adding another parameter for understanding band blade failure dynamics. The University of Siegen built and instrumented the test rig, with Albrecht Bäumer supplying the main components. The test stand allows for tests with both moving and static band blades and permits modification of the position of the twisting unit and intermediate roller supports.
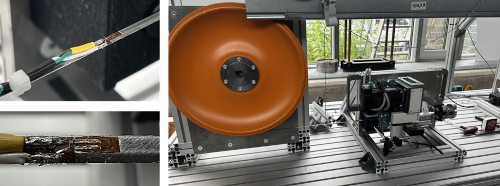